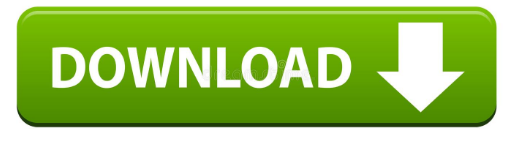
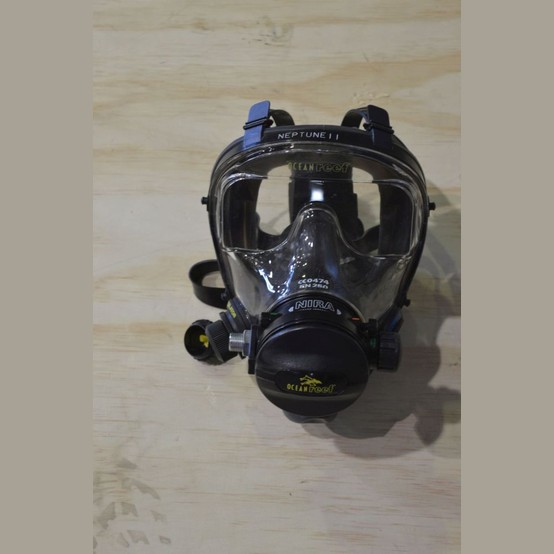
The coefficient estimate in the output indicate the average change in the log odds of the response variable associated with a one unit increase in each predictor variable.įor example, a one unit increase in the predictor variable disp is associated with an average change of -0.09518 in the log odds of the response variable am taking on a value of 1. Here’s how to interpret each piece of the output: Coefficients & P-Values Residual deviance: 16.713 on 29 degrees of freedom Null deviance: 43.230 on 31 degrees of freedom
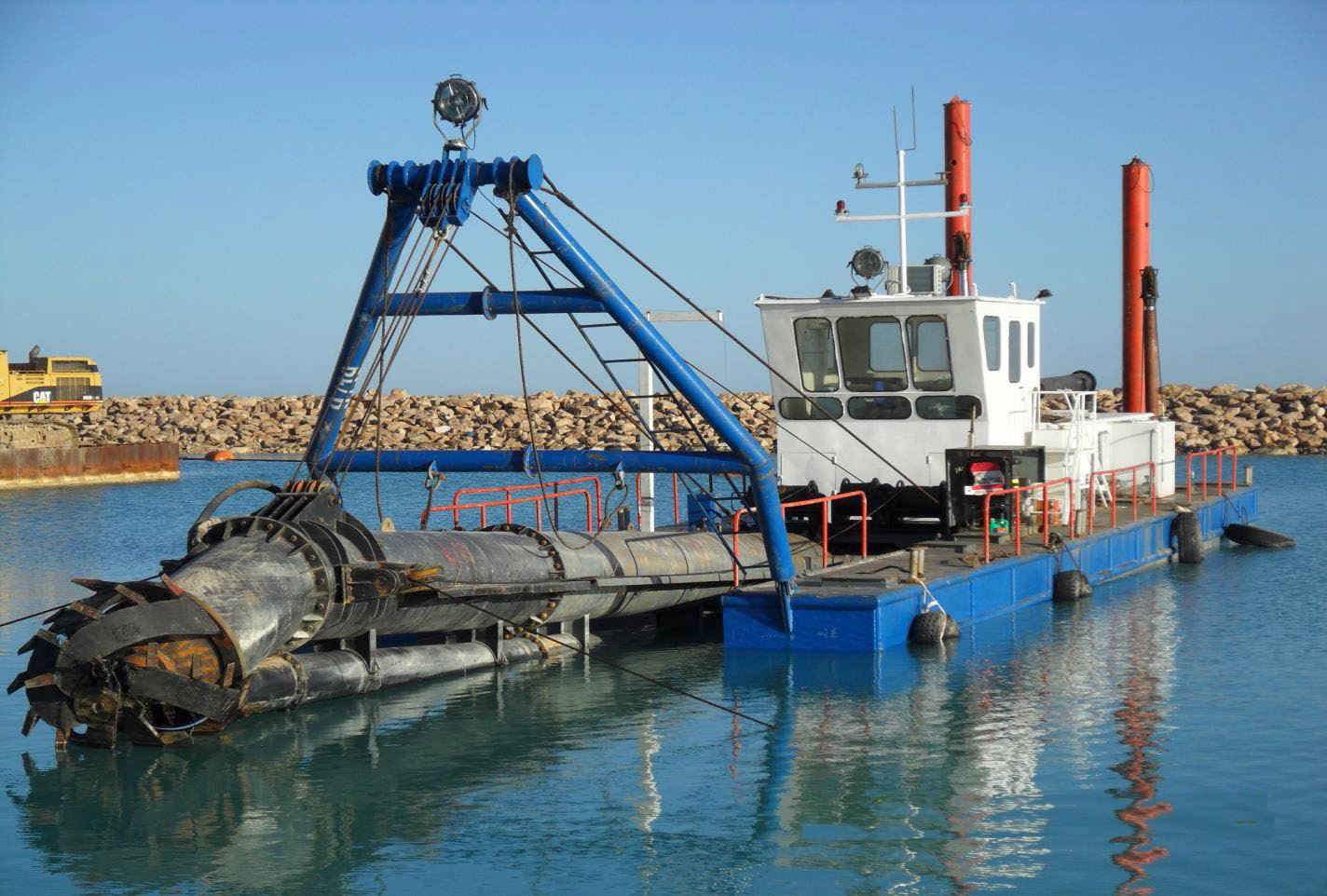
(Dispersion parameter for binomial family taken to be 1) The following code shows how to use the glm() function to fit this logistic regression model: #fit logistic regression model We will use the variables disp and hp to predict the probability that a given car takes on a value of 1 for the am variable. Mpg cyl disp hp drat wt qsec vs am gear carb Example: How to Interpret glm Output in Rįor this example, we’ll use the built-in mtcars dataset in R: #view first six rows of mtcars dataset The following example shows how to interpret the glm output in R for a logistic regression model. In practice, this function is used most often to fit logistic regression models by specifying the ‘binomial’ family. data: The name of the data frame that contains the data.Default is gaussian but other options include binomial, Gamma, and poisson among others. family: The statistical family to use to fit the model.formula: The formula for the linear model (e.g.The glm() function in R can be used to fit generalized linear models.
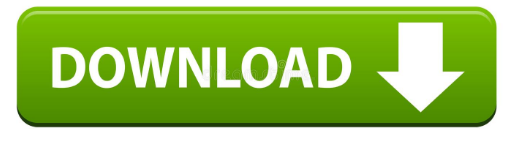